封面画师:Nengoro(ネんごろぅ) 封面ID:73408895
本文参考视频:小马哥教育(SEEMYGO) 2019年 恋上数据结构与算法(第二季)
源码仓库:mofan212/data-structure-and-algorithm (github.com)
辅助学习网址:数据结构和算法动态可视化 Data Structure Visualizations
并查集更多介绍:Union Find Algorithm
0. 需求分析
假设有 n 个村庄,有些村庄之间有连接的路,有些村庄之间并没有连接的路。
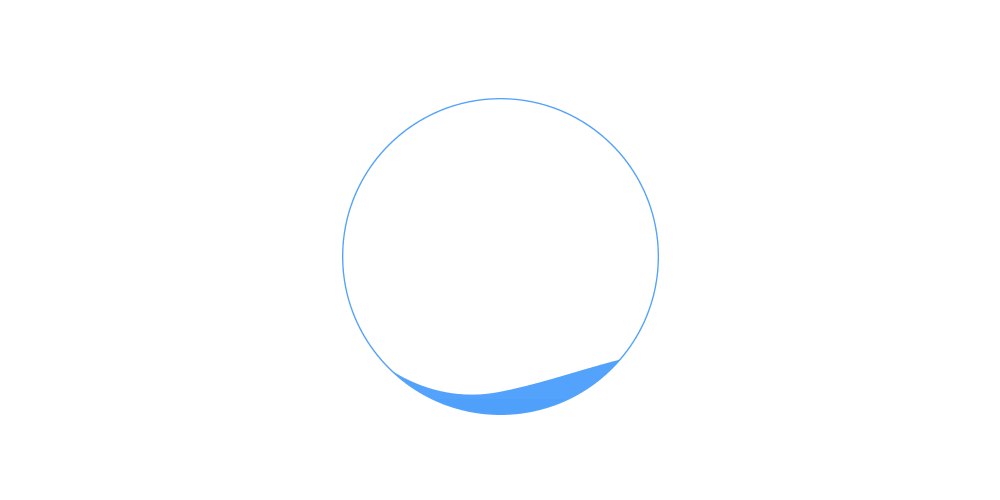
能否设计一种数据结构,能够快速执行 2 个操作:
- 查询 2 个村庄之间是否有连接的路
- 连接 2 个村庄
能否使用以前谈及的数据结构,如:数组、链表、平衡二叉树、集合(Set)等来实现呢?
其实是可以的,但是效率可能不是很好。
比如使用数组,在判断 2 个村庄之间是否有连接的路时,需要对整个村庄集聚区进行遍历,判断两个村庄是否在这个数组中,此时的时间复杂度是 O(n)。
链表和数组一样,时间复杂度也是 O(n)。
由于村庄之间不存在谁大谁小的情况,对于平衡二叉树来说,时间复杂度与数组、链表一样,也是 O(n)。
如果使用集合(HashSet
)来实现,将每个村庄聚集区看成一个HashSet
,在查询 2 个村庄之间是否连接时,只需做 O(1) 级别的查询即可,但如果每个村庄之间都没有路,相当于一个村庄就占据了一个 HashSet
,这个时候就相当于做了 O(n) 级别的查询。
如果使用以前谈及的数据结构,查询的时间复杂度都是 O(n)。
连接也是同理,时间复杂度也是 O(n)。
使用上面说到的数据结构其实有点“大材小用”的感觉,因为它们的功能并不局限于查找和连接,还有很多强大的功能,相对的底层实现也会更复杂(比如 HashSet
)。
有没有一种数据结构针对这两种需求有较好的效率,还不会出现“大材小用”呢?
并查集的查询、连接的均摊时间复杂度都是 O(α(n)),α(n)<5。
并查集非常适合解决这类“连接”相关的问题。
1. 并查集
1.1 基本概念
并查集(Union Find),也叫作不相交集合(Disjoint Set)。
并查集有两个核心操作:
- 查找(Find):查找元素所在的集合(这里的集合并不是特指
Set
这种数据结构,是指广义的数据集合)
- 合并(Union):将两个元素所在的集合合并为一个集合
并查集有两种常见的实现思路:
-
Quick Find
- 查找(Find)的时间复杂度是 O(1)
- 合并(Union)的时间复杂度是 O(n)
-
Quick Union
- 查找(Find)的时间复杂度是 O(logn),可优化至 O(α(n)),α(n)<5。
- 合并(Union)的时间复杂度是 O(logn),可优化至 O(α(n)),α(n)<5。
开发中常用的是 Quick Union。
1.2 数据存储
假设并查集处理的数据类型都是整型,那么可以用整型数组来存储数据。
数组中存储的元素就表示一个集合,相同的元素表示位于同一个集合中。
如果使用树形结构来表示,将数组中存储的元素作为父节点,每个索引按照对应的元素指向不同的父节点。比如数组中索引 0 位置的元素是 1,这表示元素 0 的父节点是 1。
我们判断数据是否位于同一个集合中,就可以判断它们的根节点是否一样。
不难看出:
- 0、1、3 属于同一个集合
- 2 单独属于一个集合
- 4、5、6、7 属于同一个集合
因此,并查集是用数组实现的树形结构,以前说过的二叉堆、优先级队列也是用数组实现的树形结构。
1.3 接口定义
1 2 3 4 5 6
| int find(int v);
void union(int v1, int v2);
boolean isSame(int v1, int v2);
|
根据提供的 find()
接口,可以很容易地编写 isSame()
的实现:
1 2 3
| public boolean isSame(int v1, int v2){ return find(v1) == find(v2); }
|
1.4 初始化
初始化时,每个元素各自属于一个单元素集合。比如:
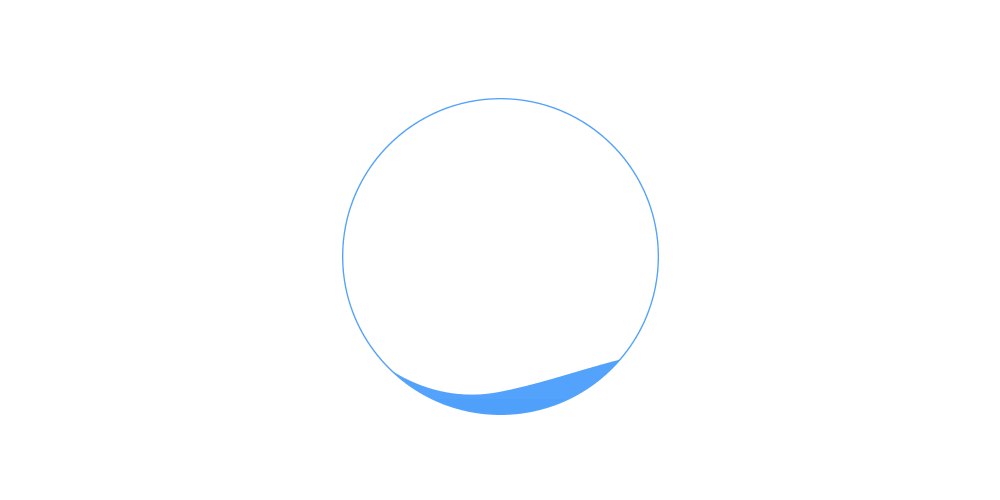
1 2 3 4 5 6 7 8 9 10 11
| private int[] parents; public UnionFind(int capacity) { if (capacity < 0) { throw new IllegalArgumentException("Capacity must >= 1."); } parents = new int[capacity]; for (int i = 0; i < parents.length; i++) { parents[i] = i; } }
|
1.5 Quick Find
对于合并方法 union(int v1, int v2)
,让 v1
所在集合的所有元素都 指向 v2
的根节点。比如:
可以发现利用这种合并方法构造出的树形结构“很平”,只有两层,数组内存储的值就是索引对应节点的根节点。
那么查找根节点 find()
接口可以这么实现:
1 2 3 4
| public int find(int v) { rangeCheck(v); return parents[v]; }
|
不难得出查找的时间复杂度是 O(1)。
对于合并来说,先查找 v1
、v2
是否在同一个集合里,即它们的根节点是否是同一个,如果是,证明它们本来就在同一个集合里,无需合并,反之,则需要将所有根节点是 v1
的节点的根节点修改为 v2
:
1 2 3 4 5 6 7 8 9 10 11
| public void union(int v1, int v2) { int p1 = find(v1); int p2 = find(v2); if (p1 == p2) return;
for (int i = 0; i < parents.length; i++) { if (parents[i] == p1) { parents[i] = p2; } } }
|
合并的时间复杂度是 O(n)。
编码实现
编写一个父类 UnionFind
,并将其定义为抽象类,以便让不同的实现继承这个父类。
1 2 3 4 5 6 7 8 9 10 11 12 13 14 15 16 17 18 19 20 21 22 23 24 25 26 27 28 29 30 31 32 33 34 35 36 37 38 39 40 41 42 43 44 45 46 47 48 49
| package com.yang.union;
public abstract class UnionFind { protected int[] parents;
public UnionFind(int capacity) { if (capacity < 0) { throw new IllegalArgumentException("capacity must be >= 1."); }
parents = new int[capacity]; for (int i = 0; i < parents.length; i++) { parents[i] = i; } }
public abstract int find(int v);
public abstract void union(int v1, int v2);
public boolean isSame(int v1, int v2) { return find(v1) == find(v2); }
protected void rangeCheck(int v) { if (v < 0 || v >= parents.length) { throw new IllegalArgumentException("v is out of bounds"); } } }
|
Quick Find 就可以这样实现:
1 2 3 4 5 6 7 8 9 10 11 12 13 14 15 16 17 18 19 20 21 22 23 24 25 26 27 28 29 30 31 32 33 34 35 36
| package com.yang.union;
public class UnionFind_QF extends UnionFind { public UnionFind_QF(int capacity) { super(capacity); }
@Override public int find(int v) { rangeCheck(v); return parents[v]; }
@Override public void union(int v1, int v2) { int p1 = find(v1); int p2 = find(v2); if (p1 == p2) return;
for (int i = 0; i < parents.length; i++){ if (parents[i] == p1){ parents[i] = p2; } } } }
|
简单测试下:
1 2 3 4 5 6 7 8 9 10 11 12 13 14 15 16 17 18 19 20 21
| public static void main(String[] args) { UnionFind uf = new UnionFind_QF(12); uf.union(0, 1); uf.union(0, 3); uf.union(0, 4); uf.union(2, 3); uf.union(2, 5);
uf.union(6, 7);
uf.union(8, 10); uf.union(9, 10); uf.union(9, 11);
System.out.println(uf.isSame(0, 6)); System.out.println(uf.isSame(0, 5)); System.out.println(uf.isSame(2, 7)); uf.union(4, 6); System.out.println(uf.isSame(2, 7)); }
|
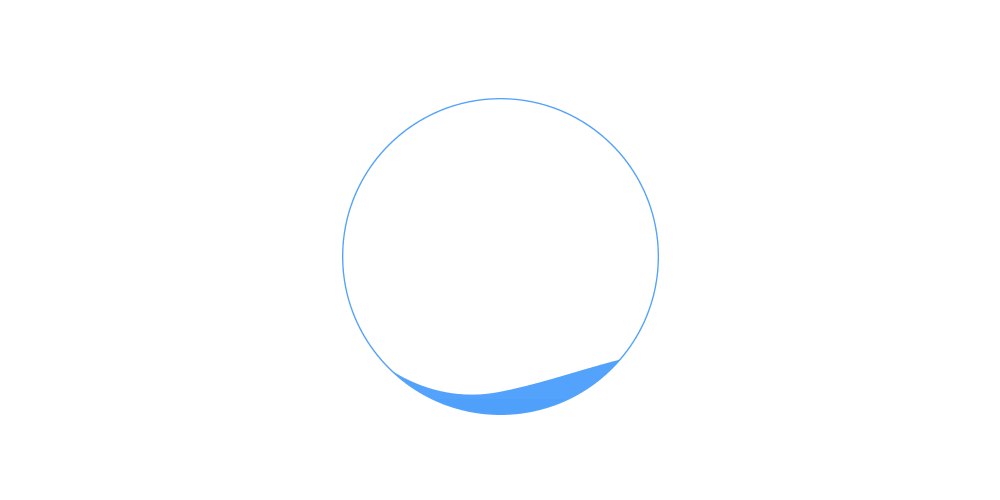
1.6 Quick Union
Union
对于合并方法 union(int v1, int v2)
,是将 v1
的 根节点 指向 v2
的根节点。注意与 Quick Find 的区别,Quick Find 是将 v1
所在集合的 所有元素 都指向 v2
的根节点。
Find
find(int v)
的作用是查找 v
所属的集合(根节点),那么对于下图所示的并查集有:
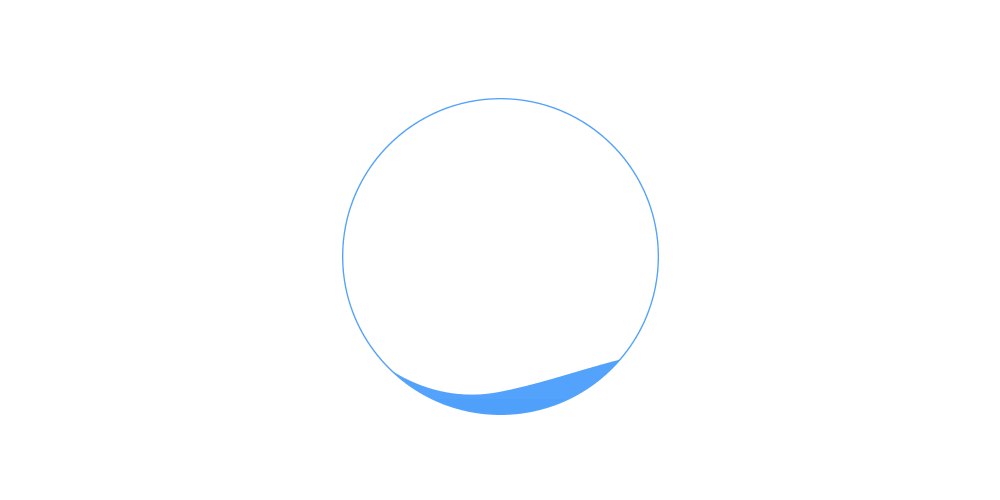
时间复杂度为 O(logn)。
一个节点的父节点等于它自身时,那么这个节点就是根节点。
1 2 3 4 5 6 7
| public int find(int v) { rangeCheck(v); while (v != parents[v]) { v = parents[v]; } return v; }
|
实现 find()
接口后,很容易实现 union()
:
1 2 3 4 5 6 7
| public void union(int v1, int v2) { int p1 = find(v1); int p2 = find(v2); if (p1 == p2) return; parents[p1] = p2; }
|
时间复杂度为 O(logn)。
编码实现
1 2 3 4 5 6 7 8 9 10 11 12 13 14 15 16 17 18 19 20 21 22 23 24 25 26 27 28 29
| public class UnionFind_QU extends UnionFind { public UnionFind_QU(int capacity) { super(capacity); }
@Override public int find(int v) { rangeCheck(v); while (v != parents[v]) { v = parents[v]; } return v; }
@Override public void union(int v1, int v2) { int p1 = find(v1); int p2 = find(v2); if (p1 == p2) return;
parents[p1] = p2; } }
|
简单测试下:
1 2 3 4 5 6 7 8 9 10 11 12 13 14 15 16 17 18 19 20 21 22 23
| public class Main { public static void main(String[] args) { UnionFind uf = new UnionFind_QU(12); uf.union(0, 1); uf.union(0, 3); uf.union(0, 4); uf.union(2, 3); uf.union(2, 5);
uf.union(6, 7);
uf.union(8, 10); uf.union(9, 10); uf.union(9, 11);
System.out.println(uf.isSame(0, 6)); System.out.println(uf.isSame(0, 5)); System.out.println(uf.isSame(2, 7)); uf.union(4, 6); System.out.println(uf.isSame(2, 7)); } }
|
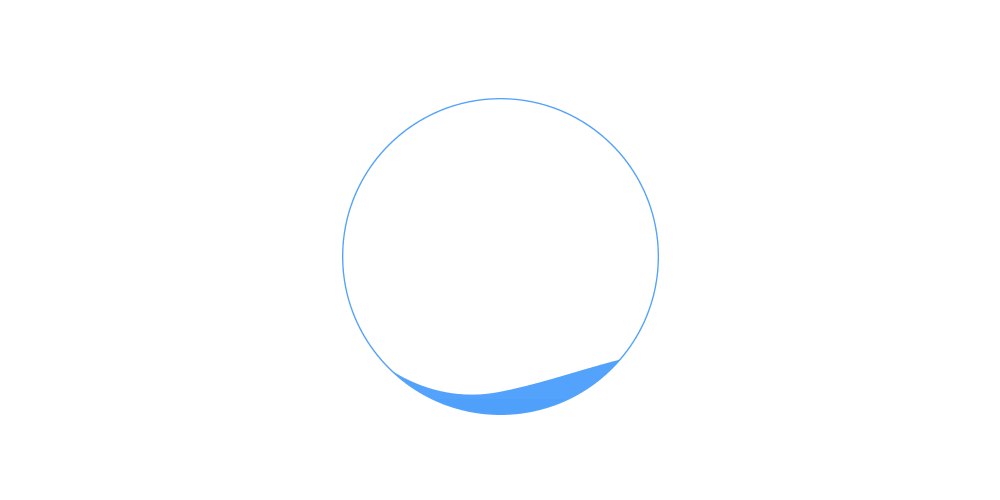
得到的测试结果与 QuickFind 一样,也证明了编码没有问题! 🎉🎊
实际应用中常用 Quick Union,因为 Quick Find 合并的时间复杂度为 O(n),实在太大,因此选用 Quick Union 作为主要实现,而且 Quick Union 还可以进一步地优化。👇
2. Quick Union优化
2.1 优化方案
在Union的过程中,可能会出现树不平衡的情况,甚至退化成链表,导致效率降低。如:
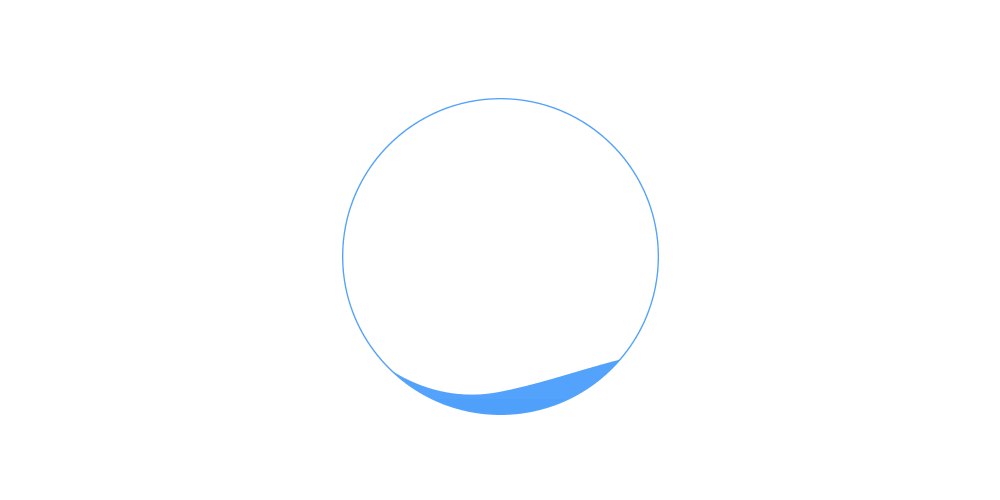
针对这个弊端,有两种常见的优化方案:
- 基于 size 的优化:元素少的树 嫁接到 元素多的树
- 基于 rank 的优化: 矮的树 嫁接到 高的树
需要注意一点,元素多少与树的高矮没有直接联系,因此上述两种方式是不同的优化方式。
2.2 基于 size 的优化
基于 size 的优化是 将元素少的树 嫁接到 元素多的树,就如同上图一样,因此需要预先知道进行合并的两棵树的元素数量,之后才方便比较并嫁接。
在构造函数中令每个集合的数量都为 1 :
1 2 3 4
| sizes = new int[capacity]; for (int i = 0; i < sizes.length; i++) { sizes[i] = 1; }
|
然后在进行合并时利用树元素的数量进行比较,查看要由哪个树嫁接到哪棵树:
1 2 3 4 5 6 7 8 9 10 11 12 13 14 15
| @Override public void union(int v1, int v2) { int p1 = find(v1); int p2 = find(v2); if (p1 == p2) return;
if (sizes[p1] < sizes[p2]) { parents[p1] = p2; sizes[p2] += sizes[p1]; } else { parents[p2] = p1; sizes[p1] += sizes[p2]; } }
|
编码实现
编写一个基于 size 的优化的 Quick Union 类,这个类继承 UnionFind_QU
,因为只有 union()
方法发生改变:
1 2 3 4 5 6 7 8 9 10 11 12 13 14 15 16 17 18 19 20 21 22 23 24 25 26 27 28 29 30 31 32 33 34 35 36
|
public class UnionFind_QU_S extends UnionFind_QU { private int[] sizes;
public UnionFind_QU_S(int capacity) { super(capacity); sizes = new int[capacity]; for (int i = 0; i < sizes.length; i++) { sizes[i] = 1; } }
@Override public void union(int v1, int v2) { int p1 = find(v1); int p2 = find(v2); if (p1 == p2) return;
if (sizes[p1] < sizes[p2]) { parents[p1] = p2; sizes[p2] += sizes[p1]; } else { parents[p2] = p1; sizes[p1] += sizes[p2]; } } }
|
实现一些用于测试的工具类
统计耗时的工具类:
1 2 3 4 5 6 7 8 9 10 11 12 13 14 15 16 17 18 19 20 21
| public class Times { private static final SimpleDateFormat fmt = new SimpleDateFormat("HH:mm:ss.SSS"); public interface Task { void execute(); } public static void test(String title, Task task) { if (task == null) return; title = (title == null) ? "" : ("【" + title + "】"); System.out.println(title); System.out.println("开始:" + fmt.format(new Date())); long begin = System.currentTimeMillis(); task.execute(); long end = System.currentTimeMillis(); System.out.println("结束:" + fmt.format(new Date())); double delta = (end - begin) / 1000.0; System.out.println("耗时:" + delta + "秒"); System.out.println("-------------------------------------"); } }
|
断言工具类:
1 2 3 4 5 6 7 8 9
| public class Asserts { public static void test(boolean value) { try { if (!value) throw new Exception("测试未通过"); } catch (Exception e) { e.printStackTrace(); } } }
|
测试使用不同的并查集实现, 8w 个随机数进行 union()
和 isSame()
所消耗的时间:
1 2 3 4 5 6 7 8 9 10 11 12 13 14 15 16 17 18 19 20 21 22 23 24 25 26 27 28 29 30 31 32 33 34 35 36 37 38 39 40 41 42 43 44 45 46
| public class Main { static final int count = 80000;
public static void main(String[] args) { test(new UnionFind_QF(12)); test(new UnionFind_QU(12)); test(new UnionFind_QU_S(12)); testTime(new UnionFind_QF(count)); testTime(new UnionFind_QU(count)); testTime(new UnionFind_QU_S(count)); }
static void test(UnionFind uf) { uf.union(0, 1); uf.union(0, 3); uf.union(0, 4); uf.union(2, 3); uf.union(2, 5);
uf.union(6, 7);
uf.union(8, 10); uf.union(9, 10); uf.union(9, 11);
Asserts.test(!uf.isSame(0, 6)); Asserts.test(uf.isSame(0, 5)); Asserts.test(!uf.isSame(2, 7)); uf.union(4, 6); Asserts.test(uf.isSame(2, 7)); }
static void testTime(UnionFind uf) { Times.test(uf.getClass().getSimpleName(), () -> { for (int i = 0; i < count; i++) { uf.union((int) (Math.random() * count), (int) (Math.random() * count)); } for (int i = 0; i < count; i++) { uf.isSame((int) (Math.random() * count), (int) (Math.random() * count)); } }); } }
|
控制台没有打印出异常信息,证明优化没有错误。
根据测试结果,就合并而言,Quick Union 的耗时 > Quick Find 的耗时 > 优化后 Quick Union 的耗时,这也证明了优化是有一定作用的。
补充拓展
虽然进行基于 size 的优化后,性能得到了明显的提升,但是依然可能会出现树不平衡的问题。
比如原本两棵树是这样的:
然后经过 union(1, 3)
就可能会出现:
这样的树依旧是不平衡的。
针对这种情况可以选择用另外一种优化方式 —— 基于 rank 的优化 。 😉
2.3 基于 rank 的优化
rank 指的是树的高度,因此基于 rank 的优化就是将矮的树嫁接到高的树上。
比如:
为了比较两棵树的高度,需要一个变量来存储树的高度,使用一个数组来进行存储。
树的高度初始化如下:
1 2 3 4
| ranks = new int[capacity]; for (int i = 0; i < ranks.length; i++) { ranks[i] = 1; }
|
编码实现
编写一个基于 rank 的优化的 Quick Union 类,这个类可以继承 UnionFind_QU
,因为只有 union()
方法发生改变:
1 2 3 4 5 6 7 8 9 10 11 12 13 14 15 16 17 18 19 20 21 22 23 24 25 26 27 28 29 30 31 32 33
|
public class UnionFind_QU_R extends UnionFind_QU { private int[] ranks;
public UnionFind_QU_R(int capacity) { super(capacity);
ranks = new int[capacity]; for (int i = 0; i < ranks.length; i++) { ranks[i] = 1; } }
public void union(int v1, int v2) { int p1 = find(v1); int p2 = find(v2); if (p1 == p2) return;
if (ranks[p1] < ranks[p2]) { parents[p1] = p2; } else if (ranks[p1] > ranks[p2]) { parents[p2] = p1; } else { parents[p1] = p2; ranks[p2] += 1; } } }
|
测试一下 Quick Union:
1 2 3 4 5 6 7 8 9
| static final int count = 500000; public static void main(String[] args) { test(new UnionFind_QF(12)); test(new UnionFind_QU(12)); test(new UnionFind_QU_S(12)); test(new UnionFind_QU_R(12)); testTime(new UnionFind_QU_S(count)); testTime(new UnionFind_QU_R(count)); }
|
测试的 数据规模增大,测试 50w 个随机数进行 union()
和 isSame()
所消耗的时间:
控制台没有打印出异常信息,证明优化没有错误。
基于 rank 的优化耗时与基于 size 的优化耗时相差不多,但前者的耗时会有一些领先,这证明优化是有作用的。
相比于基于 size 的优化,基于 rank 的优化会让树的高度相对平衡,因此 常选用基于 rank 的优化。
2.4 路径压缩
Quick Union 基于 rank 的优化演示:
虽然有了基于 rank 的优化,树会相对平衡一点,但是随着 union 次数的增多 ,树的高度依然会越来越高,导致 find 操作变慢 ,尤其是底层节点(因为 find 是不断向上寻找根节点的)。
路径压缩(Path Compression):在 find 时使路径上的所有节点都指向根节点,从而降低树的高度。比如:
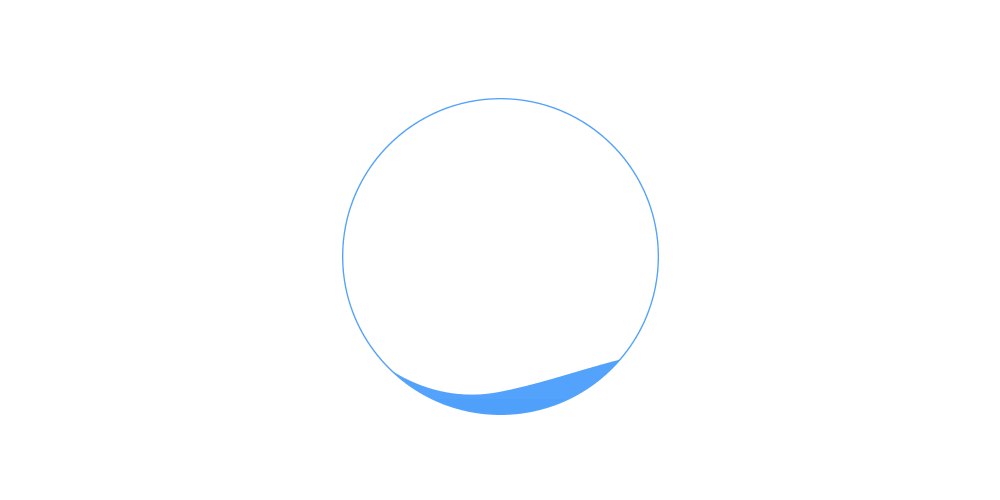
编码实现
编写一个基于 size 的优化且使用了路径压缩的 Quick Union 类,这个类继承 UnionFind_QU_R
,代码中只有 find()
方法发生改变:
1 2 3 4 5 6 7 8 9 10 11 12 13 14 15 16 17 18 19 20 21
|
public class UnionFind_QU_R_PC extends UnionFind_QU_R { public UnionFind_QU_R_PC(int capacity) { super(capacity); }
@Override public int find(int v) { rangeCheck(v);
if (parents[v] != v) { parents[v] = find(parents[v]); } return parents[v]; } }
|
测试的 数据规模增大,测试 100w 个随机数进行 union()
和 isSame()
所消耗的时间:
从测试结果中可以看出进行路径压缩后的耗时得到了减少,但是并不是很明显。这是因为在进行路径压缩时使用了递归调用,使路径上的所有节点都指向了根节点,实现成本较高。
还有两种更优的做法,不但可以降低树高,实现成本也比路径压缩更低:
- 路径分裂(Path Splitting)
- 路径减半(Path Halving)
路径分裂、路径减半的效果差不多,但都比路径压缩要好。😏
2.5 路径分裂
路径分裂(Path Splitting):使路径上的 每个节点 都指向其 祖父节点(parent 的 parent)。
进行路径分裂后的树的高度确实降低了,但是没有路径压缩那么“离谱”,并不是将所有元素都平铺开来,因此路径分裂的成本没有路径压缩的成本高。
编码实现
1 2 3 4 5 6 7 8 9 10 11 12 13 14 15 16 17 18 19 20 21
|
public class UnionFind_QU_R_PS extends UnionFind_QU_R { public UnionFind_QU_R_PS(int capacity) { super(capacity); }
@Override public int find(int v) { rangeCheck(v); while (v != parents[v]) { int p = parents[v]; parents[v] = parents[parents[v]]; v = p; } return v; } }
|
测试的 数据规模增大,测试 200w 个随机数进行 union()
和 isSame()
所消耗的时间:
相比于路径压缩,路径分裂的耗时又进一步减少了,证明路径分裂的成本没有路径压缩的成本高。
2.6 路径减半
路径减半(Path Halving):使路径上 每隔一个节点 就指向其 祖父节点(parent 的 parent)。
路径减半和路径分裂类似,进行这两种优化后得到的树的高度是一样的。
编码测试
1 2 3 4 5 6 7 8 9 10 11 12 13 14 15 16 17 18 19 20
|
public class UnionFind_QU_R_PH extends UnionFind_QU_R { public UnionFind_QU_R_PH(int capacity) { super(capacity); }
@Override public int find(int v) { rangeCheck(v); while (v != parents[v]) { parents[v] = parents[parents[v]]; v = parents[v]; } return v; } }
|
测试的 数据规模减小,测试 100w 个随机数进行 union()
和 isSame()
所消耗的时间:
路径分裂和路径减半的耗时差不多,但相比于路径压缩是更优的。
在进行优化时,可以选择路径分裂和路径减半进行优化,而不是路径压缩。
2.7 总结
摘自《维基百科》: Disjoint-set data structure
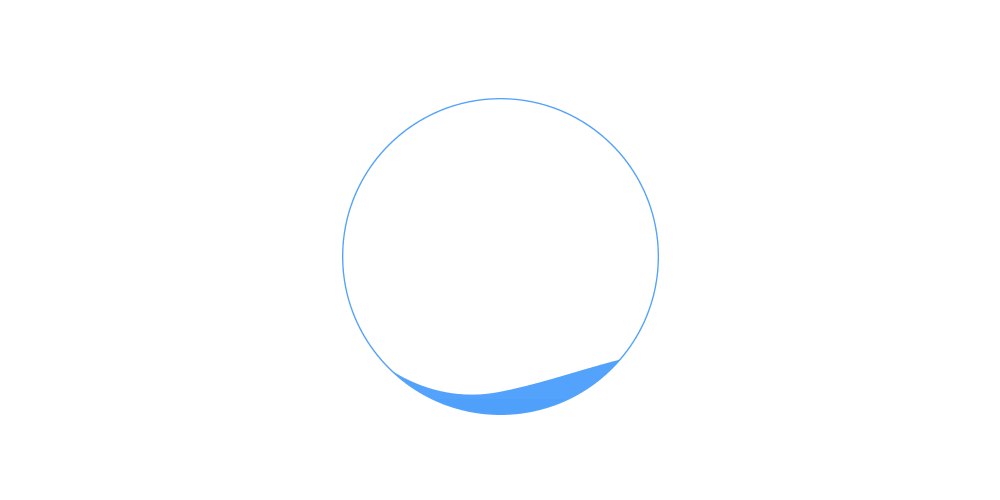
大概就是说:
使用路径压缩、分裂或减半 + 基于 rank 或者 size 的优化,可以确保每个操作的均摊时间复杂度为 O(α(n)),α(n)<5
建议的搭配
- Quick Union
- 基于 rank 的优化
- Path Halving 或 Path Splitting
3. 自定义类型
之前的使用都是基于整型数据的,如果其他自定义类型也想使用并查集应该怎么做呢?
3.1 方案一
通过一些方法将自定义类型转换为整型后使用并查集(比如生成哈希值),但转换为整型的过程的效率要高,时间复杂度最好是 O(1),如果时间复杂度达到了 O(n),那有什么意义呢?
在这种方案下,要保证不同的自定义对象生成的整型数据必须是不一样的。
总体而言,该方案是比较麻烦的。
3.2 方案二
使用链表 + 映射(Map)。
首先需要编写一个节点对象 Node
,这样数据就可以是任何类型的:
1 2 3 4 5 6 7 8 9 10
| private static class Node<V> { V value; Node<V> parent = this; int rank = 1;
public Node(V value) { this.value = value; } }
|
在这里,一个对象和一个节点对象是一一对应的,因此可以使用 Map
来存储节点:
1
| private Map<V, Node<V>> nodes = new HashMap<>();
|
在使用基于整型的并查集时,如果想使用 union(int v1, int v2)
方法是有一个前提的,就是 v1
和 v2
都是被初始化的,如:
1 2 3 4 5 6 7 8 9 10 11 12 13
| protected int[] parents;
public UnionFind(int capacity) { if (capacity < 0) { throw new IllegalArgumentException("capacity must be >= 1."); }
parents = new int[capacity]; for (int i = 0; i < parents.length; i++) { parents[i] = i; } }
|
因此基于自定义类型的并查集也需要进行初始化,可以编写一个方法对数据进行初始化:
1 2 3 4 5
| public void makeSet(V v) { if (nodes.containsKey(v)) return; nodes.put(v, new Node<>(v)); }
|
初始化完毕后,就可以实现并查集的接口了,比如:find()
、union()
、isSame()
,完整代码如下:
1 2 3 4 5 6 7 8 9 10 11 12 13 14 15 16 17 18 19 20 21 22 23 24 25 26 27 28 29 30 31 32 33 34 35 36 37 38 39 40 41 42 43 44 45 46 47 48 49 50 51 52 53 54 55 56 57 58 59 60 61 62 63
|
public class GenericUnionFind<V> { private Map<V, Node<V>> nodes = new HashMap<>();
public void makeSet(V v) { if (nodes.containsKey(v)) return; nodes.put(v, new Node<>(v)); }
private Node<V> findNode(V v) { Node<V> node = nodes.get(v); if (node == null) return null;
while (!Objects.equals(node.value, node.parent.value)) { node.parent = node.parent.parent; node = node.parent; } return node; }
public V find(V v) { Node<V> node = findNode(v); return node == null ? null : node.value; }
public void union(V v1, V v2) { Node<V> p1 = findNode(v1); Node<V> p2 = findNode(v2); if (p1 == null || p2 == null) return; if (Objects.equals(p1.value, p2.value)) return;
if (p1.rank < p2.rank) { p1.parent = p2; } else if (p1.rank > p2.rank){ p2.parent = p1; } else { p1.parent = p2; p2.rank += 1; } }
public boolean isSame(V v1, V v2) { return Objects.equals(find(v1), find(v2)); }
private static class Node<V> { V value; Node<V> parent = this; int rank = 1;
public Node(V value) { this.value = value; } } }
|
测试
编写一个自定义类型Student
:
1 2 3 4 5 6 7 8 9
| public class Student { private String name; private int age;
public Student(String name, int age) { this.name = name; this.age = age; } }
|
测试代码:
1 2 3 4 5 6 7 8 9 10 11 12 13 14 15 16 17 18 19
| public static void main(String[] args) { GenericUnionFind<Student> uf = new GenericUnionFind<>(); Student stu1 = new Student("jack", 1); Student stu2 = new Student("rose", 2); Student stu3 = new Student("jack", 3); Student stu4 = new Student("rose", 4); uf.makeSet(stu1); uf.makeSet(stu2); uf.makeSet(stu3); uf.makeSet(stu4);
uf.union(stu1, stu2); uf.union(stu3, stu4); uf.union(stu1, stu4);
Asserts.test(uf.isSame(stu2, stu3)); Asserts.test(uf.isSame(stu3, stu4)); Asserts.test(!uf.isSame(stu1, stu3)); }
|
运行后,控制台没有打印出任何异常消息,证明编写的代码无误。
既然可以使用任何类型的数据,那么使用整体的耗时是多少呢?
测试 100w 个随机数进行 union()
和 isSame()
所消耗的时间:
GenericUnionFind
的耗时是最多的,因为它可以兼容所有类型,底层还涉及了 HashMap
的使用,基于这两个原因导致 GenericUnionFind
的耗时最多。
毕竟鱼与熊掌不可兼得,又想支持任何类型,又要效率好过单一的支持,想必是很难做到的。😞